Very grateful for your pioneering work!
I want to use it in Standard Transformer released in http://nlp.seas.harvard.edu/2018/04/03/attention.html.
but it mat a mask error in training.
more detail information shown as follow, the code i use:
class ConvCompress(nn.Module):
def init(self, dim, ratio = 2, groups = 1):
super(ConvCompress, self).init()
self.conv = nn.Conv1d(dim, dim, ratio, stride = ratio, groups = groups)
#self.linear = nn.Linear(dim, dim)
def forward(self, mem):
mem = mem.transpose(1, 2)
compressed_mem = self.conv(mem)
return compressed_mem.transpose(1, 2)
class MemoryCompressedAttention(nn.Module):
def init(self, h, d_model, compression_factor = 2, dropout = 0.1):
super(MemoryCompressedAttention, self).init()
assert (d_model % h) == 0, 'dimension must be divisible by number of heads'
self.h = h
self.d_model = d_model
self.d_k = d_model // h
self.compression_factor = compression_factor
self.compress_fn = ConvCompress(d_model, compression_factor, groups = h)
#self.to_qkv = nn.Linear(dim, dim * 3, bias = False)
self.wq = nn.Linear(d_model, d_model, bias = False)
self.wk = nn.Linear(d_model, d_model, bias = False)
self.wv = nn.Linear(d_model, d_model, bias = False)
self.wo = nn.Linear(d_model, d_model)
self.dropout = nn.Dropout(dropout)
#self.null_k = nn.Parameter(torch.zeros(1, 1, d_model))
#self.null_v = nn.Parameter(torch.zeros(1, 1, d_model))
def forward(self, query, key, value, mask = None):
if mask is not None:
# Same mask applied to all h heads.
mask = mask.unsqueeze(1)
nbatches = query.size(0)
t = query.size(1)
cf = self.compression_factor
query = self.wq(query)
key = self.wk(key)
value = self.wv(value)
# make sure keys and values sequence lengths
# are divisible by the compression factor
padding = cf - (t % cf)
if padding != 0:
key, value = map(lambda t: F.pad(t, (0, 0, padding, 0)), (key, value))
# compress keys and values
key, value = map(self.compress_fn, (key, value))
# attach a null key and value, in the case that the first query has no keys to pay attention to
null_k = nn.Parameter(torch.zeros(key.size(0), 1, self.d_model)).cuda()
null_v = nn.Parameter(torch.zeros(value.size(0), 1, self.d_model)).cuda()
key = torch.cat((null_k, key), dim=1)
value = torch.cat((null_v, value), dim=1)
# merge heads
#query, key, value = map(lambda t: t.reshape(*t.shape[:2], h, -1).transpose(1, 2), (query, key, value))
# 1) Do all the linear projections in batch from d_model => h x d_k
query = query.view(nbatches, -1, self.h, self.d_k).transpose(1, 2)
key = key.view(nbatches, -1, self.h, self.d_k).transpose(1, 2)
value = value.view(nbatches, -1, self.h, self.d_k).transpose(1, 2)
# 2) Apply attention on all the projected vectors in batch.
x, self.attn = attention(query, key, value, mask=mask,
dropout=self.dropout)
# 3) "Concat" using a view and apply a final linear. # split heads and combine
x = x.contiguous().view(nbatches, -1, self.d_model)
out = self.wo(x)
return out
The error was show that
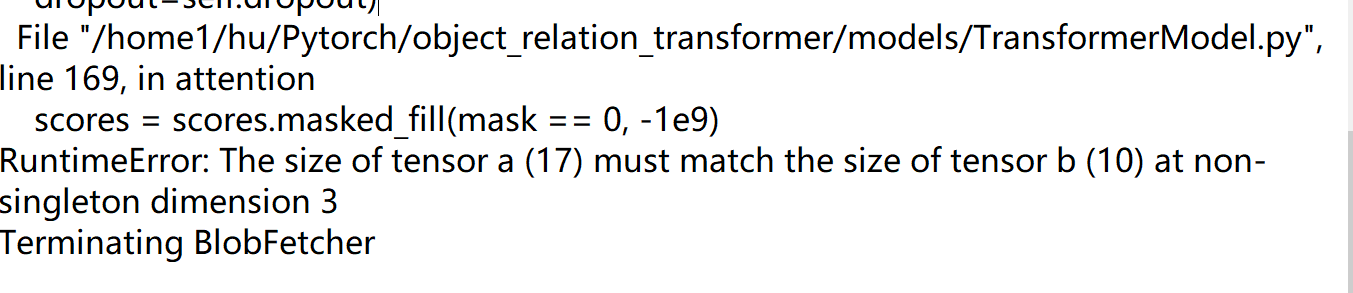
I want to know how to fix it, and how to do mask for N*M matrix??