뉴스 도메인 질의응답 시스템
본 프로젝트는 뉴스기사에 대한 질의응답 서비스
를 제공하기 위해서 진행한 프로젝트입니다. 약 3개월간 ( 21. 03 ~ 21. 05 ) 진행하였으며 Transformer 아키텍쳐 기반의 Encoder를 사용하여 한국어 질의응답 데이터셋으로 fine-tuning을 수행한 모델을 기반으로 최신 뉴스 기사를 기반으로 하여 질의응답 서비스를 제공합니다.
시스템 구성 요소 총 3가지 모듈로 구성되어 있으며 웹 클라이언트에서 질의를 입력받은 후 질문과 유사한 최신 뉴스 기사를 수집하고 이를 기반으로 기계독해를 수행하여 사용자에게 적절한 정답을 제시합니다.
웹 데모 페이지
General한 한국어 데이터셋을 기반으로 학습한 한국어 기계독해 모델에 뉴스 도메인에 적합한 추가적으로 학습한 모델을 서빙하여 실시간 질의응답 서비스를 제공한다
메인 검색 페이지
- K 지정: 관련도 최상위 K개의 문서를 리턴
- 질의 입력: 질문을 입력받음
- 검색: 검색버튼을 누르면 로딩바 재생, 검색 -> 기계독해 수행
질의 결과
- 기계독해 결과 출력: 정답이 있다고 판단한 문서에 대해서 결과출력
- 확률값을 기준으로 소팅: 확률값이 가장 높은 결과를 맨 위에 보여줌
문서 상세 보기
- 문맥 보기: 정답주변의 문맥을 볼 수 있음
- 정답 하이라이팅: 정답을 보기 쉽게 하이라이팅함
- 원본 뉴스기사 하이퍼링크: 기사 원문을 바로 찾아갈 수 있도록 제공
Requirements
For model serving
bentoml==0.12.1
torch==1.7.1
attrdict==2.0.1
fastprogress==1.0.0
numpy==1.19.2
transformers==4.1.1
scipy==1.5.4
scikit-learn==0.24.0
seqeval==1.2.2
sentencepiece==0.1.95
six==1.15.0
For web hosting
conda==4.9.2
Flask==1.1.2
html5lib @ file:///tmp/build/80754af9/html5lib_1593446221756/work
lxml @ file:///tmp/build/80754af9/lxml_1603216285000/work
MarkupSafe==1.1.1
requests @ file:///tmp/build/80754af9/requests_1592841827918/work
urllib3 @ file:///tmp/build/80754af9/urllib3_1603305693037/work
Model Serving with BentoML
두가지 MRC모델을 손쉽게 생성가능
make_single_mrc_model.py
: Threshold-based MRC Modelmake_dual_mrc_model.py
: Retrospective Reader(IntensiveReadingModule, SketchReadingModule)
모델 생성
python make_dual_mrc_model.py
모델 배포
bentoml serve DualMRCModel:latest
데이터셋
- Korquad2.0, AIHUB 기계독해 데이터셋(뉴스도메인 QAset) 사용
- Korquad2.0은 HTML태그를 제거하고 문단단위로 전처리하여 Squad2.0형식으로 변환
- Negative example을 포함하여 변환된 Korquad2.0 데이터 셋120만개와 AIHUB 기계독해 데이터셋 28만개를 학습시 사용
- 약 7만개의 AIHUB 기계독해 데이터셋을 평가시 사용
모델 학습
- 코쿼드 데이터를 3번, AIHUB 데이터를 7번 반복학습
- 파라미터는 KoELECTRA-small-v3 모델의 configuration을 그대로 사용
모델 평가
General
- 변환한 코쿼드의 데브셋 약 13만개를 평가 데이터로 사용
- Soft/Hard 필터링 모델에 대한 평가 수행
Soft 필터링
- Retrospective Reader 구조를 한국어 기계독해에 적용
- SketchReading, IntensiveReading의 정보를 합산하여 정답을 검증
- 가중치 변수는 추론 정보의 조합 비율을 말함
- 아래와 같이 두 가지 모듈의 정보를 적절히 반영했을때 NoAnswer 분류 성능이 더 좋음을 알 수 있었음
Hard 필터링
- 문단별 선별적으로 독해하는 상황을 가정함
- SketchReading에서 정답이 없다고 판별한 경우 과감히 Skip
- 추론 효율 향상과 정답이 없는 문단을 독해하여 발생할 수 있는 Negative bias를 줄이고자 함.
- 하지만 필터링 비율에 따라서 성능저하 발생
- 따라서, Positive example의 추론여부가 중요한 기계독해에선 Soft필터링 방식이 적절함을 보임 <<<<<<< HEAD
=======
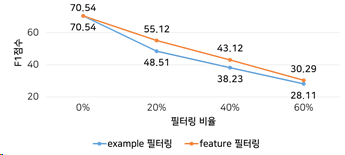
2317d0e137e1d25027ee78b55df4e5682a391295
Domain-Specific
- AIHUB 기계독해 데이터셋 35만개의 일부를(20%) 평가 데이터로 사용
- 단일 모델에 대한 평가만 수행
- NoAnswer 분류시 사용하는 임계값을 변경하며 실험
Total (EM) |
Total (F1) |
정답이 있는 경우 (F1) |
정답이 없는 경우 (acc) |
|
---|---|---|---|---|
KoreanNewsQAModel | X | X | 81.84 | X |
KoreanNewsQAModel(th=10) | 67.87 | 82.56 | 81.64 | 84.85 |
KoreanNewsQAModel(th=0) | 70.58 | 84.92 | 80.53 | 95.89 |
전체적인 성능치를 고려하여 임계값을 0으로 설정하여 모델을 서빙하기로 결정
Citation
@misc{park2020koelectra,
author = {Park, Jangwon},
title = {KoELECTRA: Pretrained ELECTRA Model for Korean},
year = {2020},
publisher = {GitHub},
journal = {GitHub repository},
howpublished = {\url{https://github.com/monologg/KoELECTRA}}
}