deepface
Deepface is a lightweight face recognition and facial attribute analysis (age, gender, emotion and race) framework for python. It is a hybrid face recognition framework wrapping state-of-the-art models: VGG-Face
, Google FaceNet
, OpenFace
, Facebook DeepFace
, DeepID
, ArcFace
and Dlib
.
Experiments show that human beings have 97.53% accuracy on facial recognition tasks whereas those models already reached and passed that accuracy level.
Installation
The easiest way to install deepface is to download it from PyPI
. It's going to install the library itself and its prerequisites as well. The library is mainly powered by TensorFlow and Keras.
pip install deepface
Then you will be able to import the library and use its functionalities.
from deepface import DeepFace
Facial Recognition - Demo
A modern face recognition pipeline consists of 5 common stages: detect, align, normalize, represent and verify. While Deepface handles all these common stages in the background, you don’t need to acquire in-depth knowledge about all the processes behind it. You can just call its verification, find or analysis function with a single line of code.
Face Verification - Demo
This function verifies face pairs as same person or different persons. It expects exact image paths as inputs. Passing numpy or based64 encoded images is also welcome. Then, it is going to return a dictionary and you should check just its verified key.
result = DeepFace.verify(img1_path = "img1.jpg", img2_path = "img2.jpg")
Face recognition - Demo
Face recognition requires applying face verification many times. Herein, deepface has an out-of-the-box find function to handle this action. It's going to look for the identity of input image in the database path and it will return pandas data frame as output.
df = DeepFace.find(img_path = "img1.jpg", db_path = "C:/workspace/my_db")
Face recognition models - Demo
Deepface is a hybrid face recognition package. It currently wraps many state-of-the-art face recognition models: VGG-Face
, Google FaceNet
, OpenFace
, Facebook DeepFace
, DeepID
, ArcFace
and Dlib
. The default configuration uses VGG-Face model.
models = ["VGG-Face", "Facenet", "Facenet512", "OpenFace", "DeepFace", "DeepID", "ArcFace", "Dlib"]
#face verification
result = DeepFace.verify(img1_path = "img1.jpg", img2_path = "img2.jpg", model_name = models[1])
#face recognition
df = DeepFace.find(img_path = "img1.jpg", db_path = "C:/workspace/my_db", model_name = models[1])
FaceNet, VGG-Face, ArcFace and Dlib are overperforming ones based on experiments. You can find out the scores of those models below on both Labeled Faces in the Wild and YouTube Faces in the Wild data sets declared by its creators.
Model | LFW Score | YTF Score |
---|---|---|
Facenet512 | 99.65% | - |
ArcFace | 99.41% | - |
Dlib | 99.38 % | - |
Facenet | 99.20% | - |
VGG-Face | 98.78% | 97.40% |
Human-beings | 97.53% | - |
OpenFace | 93.80% | - |
DeepID | - | 97.05% |
Similarity
Face recognition models are regular convolutional neural networks and they are responsible to represent faces as vectors. We expect that a face pair of same person should be more similar than a face pair of different persons.
Similarity could be calculated by different metrics such as Cosine Similarity, Euclidean Distance and L2 form. The default configuration uses cosine similarity.
metrics = ["cosine", "euclidean", "euclidean_l2"]
#face verification
result = DeepFace.verify(img1_path = "img1.jpg", img2_path = "img2.jpg", distance_metric = metrics[1])
#face recognition
df = DeepFace.find(img_path = "img1.jpg", db_path = "C:/workspace/my_db", distance_metric = metrics[1])
Euclidean L2 form seems to be more stable than cosine and regular Euclidean distance based on experiments.
Facial Attribute Analysis - Demo
Deepface also comes with a strong facial attribute analysis module including age
, gender
, facial expression
(including angry, fear, neutral, sad, disgust, happy and surprise) and race
(including asian, white, middle eastern, indian, latino and black) predictions.
obj = DeepFace.analyze(img_path = "img4.jpg", actions = ['age', 'gender', 'race', 'emotion'])
Age model got ± 4.65 MAE; gender model got 97.44% accuracy, 96.29% precision and 95.05% recall as mentioned in its tutorial.
Streaming and Real Time Analysis - Demo
You can run deepface for real time videos as well. Stream function will access your webcam and apply both face recognition and facial attribute analysis. The function starts to analyze a frame if it can focus a face sequantially 5 frames. Then, it shows results 5 seconds.
DeepFace.stream(db_path = "C:/User/Sefik/Desktop/database")
Even though face recognition is based on one-shot learning, you can use multiple face pictures of a person as well. You should rearrange your directory structure as illustrated below.
user
├── database
│ ├── Alice
│ │ ├── Alice1.jpg
│ │ ├── Alice2.jpg
│ ├── Bob
│ │ ├── Bob.jpg
Face Detectors - Demo
Face detection and alignment are important early stages of a modern face recognition pipeline. Experiments show that just alignment increases the face recognition accuracy almost 1%. OpenCV
, SSD
, Dlib
, MTCNN
, RetinaFace
and MediaPipe
detectors are wrapped in deepface.
All deepface functions accept an optional detector backend input argument. You can switch among those detectors with this argument. OpenCV is the default detector.
backends = ['opencv', 'ssd', 'dlib', 'mtcnn', 'retinaface', 'mediapipe']
#face verification
obj = DeepFace.verify(img1_path = "img1.jpg", img2_path = "img2.jpg", detector_backend = backends[4])
#face recognition
df = DeepFace.find(img_path = "img.jpg", db_path = "my_db", detector_backend = backends[4])
#facial analysis
demography = DeepFace.analyze(img_path = "img4.jpg", detector_backend = backends[4])
#face detection and alignment
face = DeepFace.detectFace(img_path = "img.jpg", target_size = (224, 224), detector_backend = backends[4])
Face recognition models are actually CNN models and they expect standard sized inputs. So, resizing is required before representation. To avoid deformation, deepface adds black padding pixels according to the target size argument after detection and alignment.
RetinaFace and MTCNN seem to overperform in detection and alignment stages but they are much slower. If the speed of your pipeline is more important, then you should use opencv or ssd. On the other hand, if you consider the accuracy, then you should use retinaface or mtcnn.
The performance of RetinaFace is very satisfactory even in the crowd as seen in the following illustration. Besides, it comes with an incredible facial landmark detection performance. Highlighted red points show some facial landmarks such as eyes, nose and mouth. That's why, alignment score of RetinaFace is high as well.
You can find out more about RetinaFace on this repo.
API - Demo
Deepface serves an API as well. You can clone /api/api.py
and pass it to python command as an argument. This will get a rest service up. In this way, you can call deepface from an external system such as mobile app or web.
python api.py
Face recognition, facial attribute analysis and vector representation functions are covered in the API. You are expected to call these functions as http post methods. Service endpoints will be http://127.0.0.1:5000/verify
for face recognition, http://127.0.0.1:5000/analyze
for facial attribute analysis, and http://127.0.0.1:5000/represent
for vector representation. You should pass input images as base64 encoded string in this case. Here, you can find a postman project.
Face recognition models represent facial images as vector embeddings. The idea behind facial recognition is that vectors should be more similar for same person than different persons. The question is that where and how to store facial embeddings in a large scale system. Herein, deepface offers a represention function to find vector embeddings from facial images.
embedding = DeepFace.represent(img_path = "img.jpg", model_name = 'Facenet')
Tech stack is vast to store vector embeddings. To determine the right tool, you should consider your task such as face verification or face recognition, priority such as speed or confidence, and also data size.
Contribution
Pull requests are welcome. You should run the unit tests locally by running test/unit_tests.py
. Please share the unit test result logs in the PR. Deepface is currently compatible with TF 1 and 2 versions. Change requests should satisfy those requirements both.
Support
There are many ways to support a project - starring
You can also support this work on Patreon
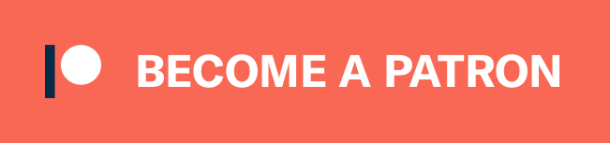
Citation
Please cite deepface in your publications if it helps your research. Here are BibTeX entries:
@inproceedings{serengil2020lightface,
title = {LightFace: A Hybrid Deep Face Recognition Framework},
author = {Serengil, Sefik Ilkin and Ozpinar, Alper},
booktitle = {2020 Innovations in Intelligent Systems and Applications Conference (ASYU)},
pages = {23-27},
year = {2020},
doi = {10.1109/ASYU50717.2020.9259802},
url = {https://doi.org/10.1109/ASYU50717.2020.9259802},
organization = {IEEE}
}
@inproceedings{serengil2021lightface,
title = {HyperExtended LightFace: A Facial Attribute Analysis Framework},
author = {Serengil, Sefik Ilkin and Ozpinar, Alper},
booktitle = {2021 International Conference on Engineering and Emerging Technologies (ICEET)},
pages = {1-4},
year = {2021},
doi = {10.1109/ICEET53442.2021.9659697},
url = {https://doi.org/10.1109/ICEET53442.2021.9659697},
organization = {IEEE}
}
Also, if you use deepface in your GitHub projects, please add deepface in the requirements.txt.
Licence
Deepface is licensed under the MIT License - see LICENSE
for more details. However, the library wraps some external face recognition models: VGG-Face, Facenet, OpenFace, DeepFace, DeepID, ArcFace and Dlib. Besides, age, gender and race / ethnicity models are based on VGG-Face. Licence types will be inherited if you are going to use those models. Please check the license types of those models for production purposes.
Deepface logo is created by Adrien Coquet and it is licensed under Creative Commons: By Attribution 3.0 License.