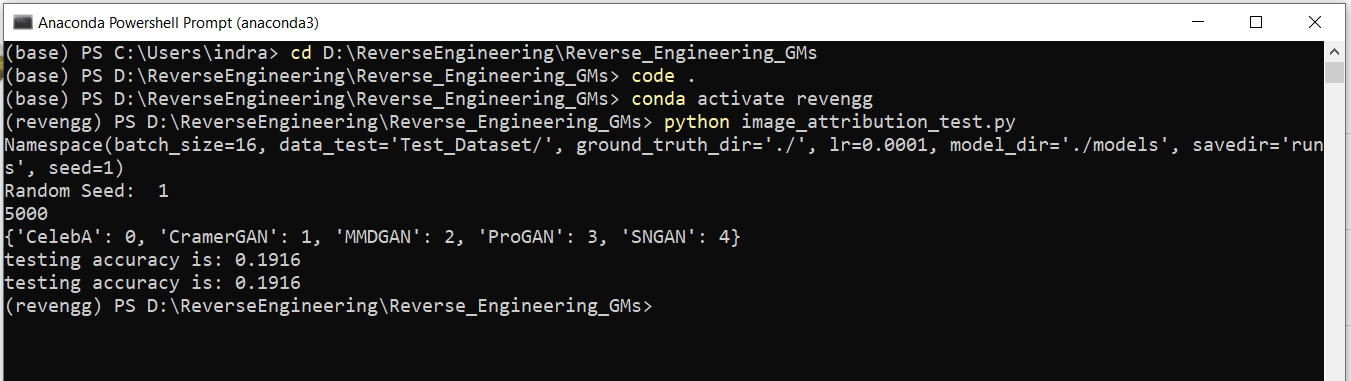
I'm getting only 0.1916 accuracy in image attribution task, in the test dataset in each of the five classes I've puted 1K generated images from respective GANs and 1K real images from CelebA, and I'm using the pre-trained model.
I'm using the following code in image_attribution_test.py file:
from torchvision import datasets, models, transforms
#from model import *
import os
import torch
from torch.autograd import Variable
from skimage import io
from scipy import fftpack
import numpy as np
from torch import nn
import datetime
from models import encoder_image_attr
from models import fen
import torch.nn.functional as F
from sklearn.metrics import accuracy_score
from sklearn import metrics
import argparse
parser = argparse.ArgumentParser()
parser.add_argument('--lr', default=0.0001, type=float, help='learning rate')
parser.add_argument('--data_test',default='Test_Dataset/',help='root directory for testing data')
parser.add_argument('--ground_truth_dir',default='./',help='directory for ground truth')
parser.add_argument('--seed', default=1, type=int, help='manual seed')
parser.add_argument('--batch_size', default=16, type=int, help='batch size')
parser.add_argument('--savedir', default='runs')
parser.add_argument('--model_dir', default='./models')
opt = parser.parse_args()
print(opt)
print("Random Seed: ", opt.seed)
device=torch.device("cuda:0")
torch.backends.deterministic = True
torch.manual_seed(opt.seed)
torch.cuda.manual_seed_all(opt.seed)
sig = "sig"
test_path=opt.data_test
save_dir=opt.savedir
os.makedirs('%s/logs/%s' % (save_dir, sig), exist_ok=True)
os.makedirs('%s/result_2/%s' % (save_dir, sig), exist_ok=True)
transform_train = transforms.Compose([
transforms.Resize((128,128)),
transforms.ToTensor(),
transforms.Normalize((0.6490, 0.6490, 0.6490), (0.1269, 0.1269, 0.1269))
])
test_set=datasets.ImageFolder(test_path, transform_train)
test_loader = torch.utils.data.DataLoader(test_set,batch_size=opt.batch_size,shuffle =True, num_workers=1)
model=fen.DnCNN().to(device)
model_params = list(model.parameters())
optimizer = torch.optim.Adam(model_params, lr=opt.lr)
l1=torch.nn.MSELoss().to(device)
l_c = torch.nn.CrossEntropyLoss().to(device)
model_2=encoder_image_attr.encoder(num_hidden=512).to(device)
optimizer_2 = torch.optim.Adam(model_2.parameters(), lr=opt.lr)
state = {
'state_dict_cnn':model.state_dict(),
'optimizer_1': optimizer.state_dict(),
'state_dict_class':model_2.state_dict(),
'optimizer_2': optimizer_2.state_dict()
}
state1 = torch.load("pre_trained_models/image_attribution/celeba/0_model_27_384000.pickle")
optimizer.load_state_dict(state1['optimizer_1'])
model.load_state_dict(state1['state_dict_cnn'])
optimizer_2.load_state_dict(state1['optimizer_2'])
model_2.load_state_dict(state1['state_dict_class'])
def test(batch, labels):
model.eval()
model_2.eval()
with torch.no_grad():
y,low_freq_part,max_value ,y_orig,residual, y_trans,residual_gray =model(batch.type(torch.cuda.FloatTensor))
y_2=torch.unsqueeze(y.clone(),1)
classes, features=model_2(y_2)
classes_f=torch.max(classes, dim=1)[0]
n=25
zero=torch.zeros([y.shape[0],2*n+1,2*n+1], dtype=torch.float32).to(device)
zero_1=torch.zeros(residual_gray.shape, dtype=torch.float32).to(device)
loss1=0.5*l1(low_freq_part,zero).to(device)
loss2=-0.001*max_value.to(device)
loss3 = 0.01*l1(residual_gray,zero_1).to(device)
loss_c =10*l_c(classes,labels.type(torch.cuda.LongTensor))
loss5=0.1*l1(y,y_trans).to(device)
loss=(loss1+loss2+loss3+loss_c+loss5)
return y, loss.item(), loss1.item(),loss2.item(),loss3.item(),loss_c.item(),loss5.item(),y_orig, features,residual,torch.max(classes, dim=1)[1], classes[:,1]
print(len(test_set))
print(test_set.class_to_idx)
epochs=2
for epoch in range(epochs):
all_y=[]
all_y_test=[]
flag1=0
count=0
itr=0
for batch_idx_test, (inputs_test,labels_test) in enumerate(test_loader):
out,loss,loss1,loss2,loss3,loss4,loss5, out_orig,features,residual,pred,scores=test(Variable(torch.FloatTensor(inputs_test)),Variable(torch.LongTensor(labels_test)))
if flag1==0:
all_y_test=labels_test
all_y_pred_test=pred.detach()
all_scores=scores.detach()
flag1=1
else:
all_y_pred_test=torch.cat([all_y_pred_test,pred.detach()], dim=0)
all_y_test=torch.cat([all_y_test,labels_test], dim=0)
all_scores=torch.cat([all_scores,scores], dim=0)
fpr1, tpr1, thresholds1 = metrics.roc_curve(all_y_test, np.asarray(all_scores.cpu()), pos_label=1)
print("testing accuracy is:", accuracy_score(all_y_test,np.asarray(all_y_pred_test.cpu())))